Blog
Generative AI adoption risks you should address to safeguard your enterprise
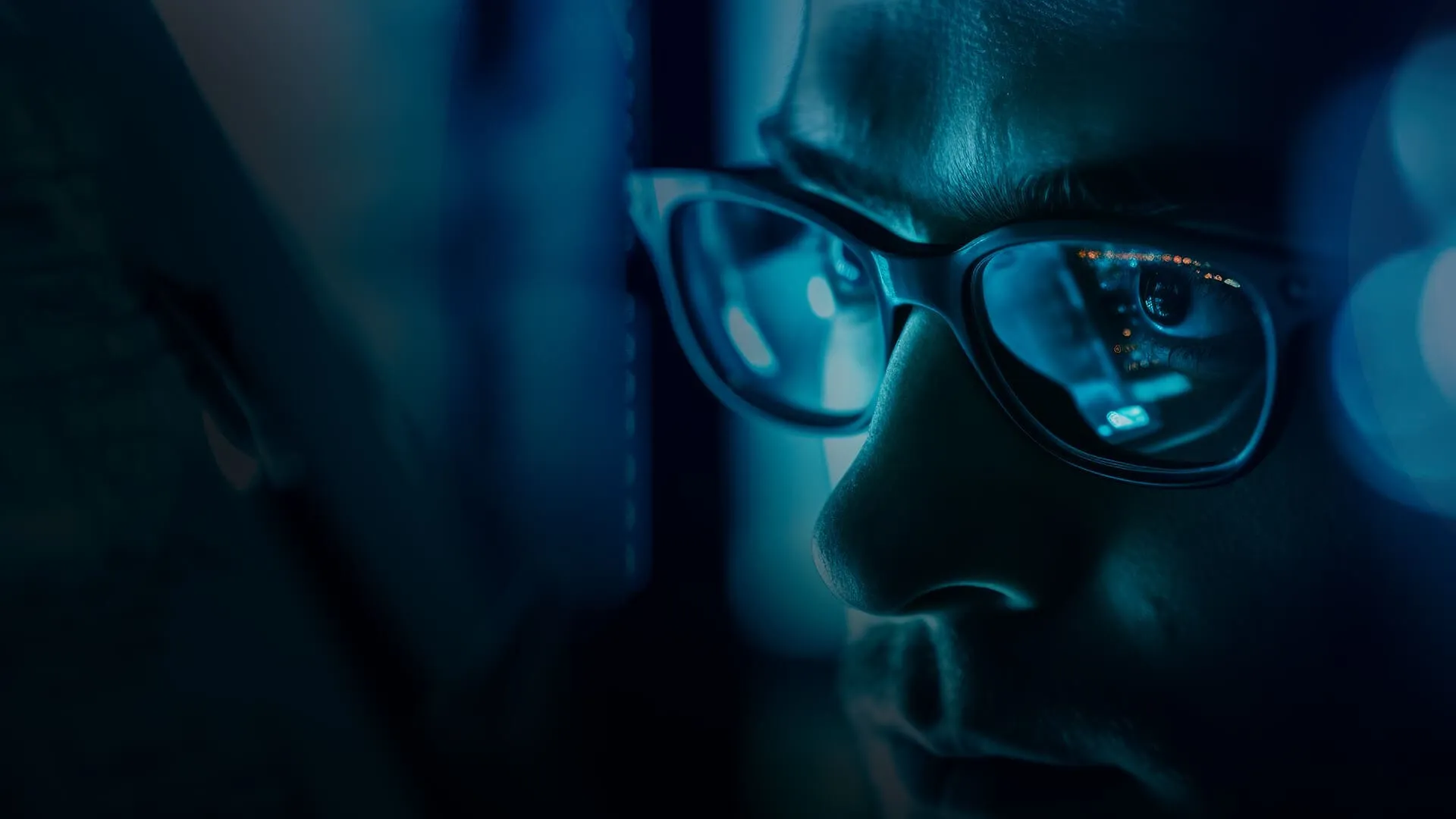
Generative AI’s remarkable ability to analyze large volumes of data quickly, learn from existing datasets, and create new content (text, images, audio, video) continues to create varied use cases across industries. These include customer support, marketing content creation, health diagnostics, software code generation, business performance analytics etc. Across industry sectors, enterprises are therefore understandably keen to quickly harness the transformative potential of this disruptive technology. However, business and technology leaders need to exercise caution when embracing Generative AI.
At this stage of their evolution, Generative AI tools are useful as decision support tools. However, they should not be relied on for decision-making without human experts regularly validating the output. Also, in the absence of adequate safeguards, Generative AI adoption can cause cybersecurity risks, data privacy risks, intellectual property (IP) risks etc.
The inadequacy of safeguards is a consequence of both lack of awareness of how Generative AI models and tools work, as also pressure to deploy Generative AI quickly. These lacunae have serious implications for enterprise information security. Examples of employees unwittingly uploaded sensitive code onto ChatGPT have forced enterprises to take extreme actions like banning the use of such Generative AI platforms altogether. But that is not a sustainable solution because Generative AI is here to stay.
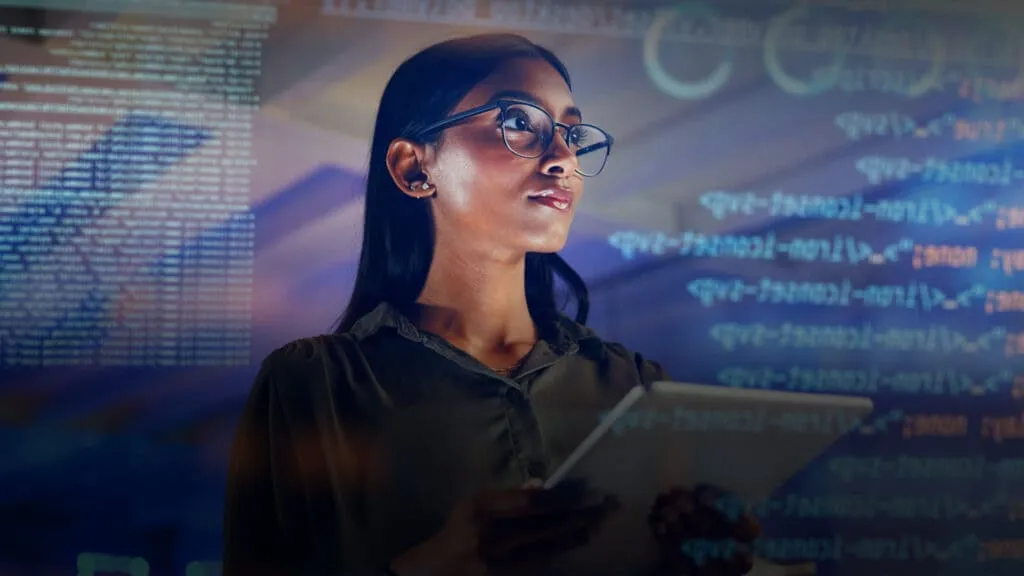
Business and technology leaders must be aware of Generative AI adoption threats
The combination of rapid digitalization and the increasing pervasiveness of Generative AI obviously raise the specter of cybersecurity threats. Bad actors are already using Generative AI tools to create more authentic-looking websites and fake messages to bait consumers; they are using voice samples taken from various sources to engineer credible impersonations. All this is leading to the spread of misinformation and increasingly sophisticated phishing attacks and frauds at scale.
CISOs and other business and technology leaders need to be concerned about the multiple risks associated with Generative AI adoption. These risks pertain to cybersecurity, risks associated with bias and hallucination, as well as risks related to leaking of confidential information and/or IP violation. Collectively, these risks can damage the performance of enterprise by blunting their competitive edge and causing damaging to the brand/reputation.
Listed below are the five major risks associated with Generative AI that enterprises must be aware of so that they can consciously address them.
-
Confidential or sensitive data going public:
- Any information (including snippets of code, creative content, plans, customer data etc.) that employees feed into a public AI LLM (Large Language Model) like ChatGPT goes into the public domain. Others can legitimately gain access it through appropriate prompts. Besides, hackers can break into these LLMs. Access to LLMs may be banned at workplaces, but what happens if employees working from home engage in such behavior?
-
Data privacy:
- Rules pertaining to data privacy and protection are getting more stringent by the day; different countries are coming up with their own regulations. Enterprises face compliance risks if “Personally Identifiable Information” gets inadvertently shared with third-party AI solution providers (or is leaked by hackers). This risk is amplified if APIs used to access customer data repositories are exposed.
-
Data poisoning:
- LLMs are trained through ingestion of hundreds of millions of tokens over a period of time. During this phase, adversaries may seek to deliberately “poison” the datasets used to train LLMs. Such malicious actions can lead to incorrect outcomes at a later stage, thus impacting the quality of decisions made. Third parties too can exploit vulnerabilities and lack of encryption/controls in LLMs and associated modules to steal data.
-
Intellectual Property:
- LLMs cannot identify and attribute IP rights. As a result, enterprises may inadvertently may end up violating someone else’s legally-protected IP. For example, Stable Diffusion has used millions of images to train its LLMs. Getty Images, which owns the IP rights to some of these images, has filed a lawsuit. Other creative work such as paintings, songs, poetry, books, plays, software code, business plan formats, methodologies etc. available on the internet have been used to train various LLMs. As the owners of the underlying IPR have not explicitly provided consent to LLM owners/users to use their IP, there is a rise in the number of lawsuits against companies building LLMs. Enterprises using these LLMs run the risk of being included in such lawsuits.
-
Lack of robust Generative AI governance mechanism:
- As enterprises embrace Generative AI, there may be multiple projects underway. In the absence of a suitable unified approach, different projects may create different kinds of risk, making an already complex challenge even more difficult to address.
Enterprises must prepare to face Generative AI security, data privacy, IP violation/leak, and bias threats
Going forward, all business enterprises will encounter more AI-powered cyberattacks from an increasing number of sources using sophisticated attack vectors. These threats can manifest when enterprises are:
- using output generated by Generative AI systems;
- developing and deploying defence capabilities to identify vulnerabilities, detect threats, and respond to incidents with greater speed and effectiveness; and/or
- building in-house Generative AI applications and integrating them with third-party software and platforms.
The different threats described in the preceding section have the potential to be triggered during the above activities.
A Gartner study reveals that “89% of business technologists would bypass cybersecurity guidance to meet a business objective”. Information security is thus not just the responsibility of CISOs. Every employee is responsible to safeguard the enterprise. This needs a fundamental shift in mindsets and ways of working. Enterprises will need to transform the way their business and technology teams collaborate, as well as how devops teams and SOCs are organized and function. Technical capabilities and automation alone will not suffice; all stakeholders must consciously work together to ensure that there is accountability for information security at all levels.
Robust governance is critical to successful Generative AI adoption
Enterprises must put in place a robust governance mechanism to ensure that AI projects are envisioned, designed, implemented, and managed in ways that minimize cybersecurity risks. The various facets of such governance must include anonymization of data, training employees, vetting Generative AI tools selected, regular vendor audits etc. Periodic reviews of processes, vendors, etc. must be part of the overall governance framework so that necessary changes can be made in the light of new threats, emerging vulnerabilities, and experiences drawn from actual incidents of breach. All this must be done in a unified, seamless way, across functional/project teams, departments, and geographies.
To understand the specific Generative AI related security risks in your enterprise and explore how to address them, write to us on [email protected]. We will schedule a commitment-free discussion with our experts, who will be happy to suggest tangible actions to safeguard your business. For more information about our company, visit https://paramountassure.com/.
ABOUT AUTHOR
Recent Posts
- Achieving Zero Trust in the Middle East with Endpoint Privilege Management
- Let’s root for each other and watch each other grow
- Best Practices for Implementing Robust OT Security
- Maximize Cybersecurity with Existing Cloud Investments | Paramount
- Cloud and BYOD – Securely Integrating for Business Growth

Protect your online assets from cyber threats with Paramount

Comprehensive cyber security solutions for individuals and businesses

Significantly reduce the risk of cyber threats and ensure a safer digital environment.